This article is the second in a series about AI and how you can begin to think about and leverage the various AI technologies. Last time we talked about AI myths and the different ways it can be used in its current state to help drive efficiency in your business. Today we are going to take a closer look at the importance of data as it pertains to AI-driven solutions. Like any system, you get out what you put in. I’m Scott Geosits, CTO here at Trifecta, and I’ll walk you through it.
Good data is the lifeblood of building and training effective AI models, and plays a vital role in their reliability and ethical considerations. It influences every aspect of AI model development, from accuracy and fairness to ethical considerations and regulatory compliance. To harness the full potential of AI and minimize its risks, it is essential to prioritize the collection, curation, and management of high-quality data throughout the AI development lifecycle. But what does “good data” actually mean?
When thinking about data for AI models, we want to focus on data that meets certain criteria – making it valuable and reliable for analysis, decision-making and other purposes.
Good data should meet the following criteria:
- Accurate – free from errors, inaccuracies, and inconsistencies. Should reflect the true and current state of the information it represents
- Complete – contains all the relevant information needed for an outcome, and is not missing important values or attributes
- Consistent – data is uniform and follows the same format, units and standards throughout a dataset
- Timely – up to date and relevant for the task at hand
- Relevant – pertinent to the specific problem or question being addressed
- Reliable – consistent and trustworthy, and can be depended on to produce consistent results. Also collected, stored, and managed in a reliable manner
- Valid – represents the concepts it is intended to measure or describe
- Precise – the level of detail in the data – should be precise enough to fulfill the intended purpose without being unnecessarily granular and complex
- Bias-Free – collected and managed without any biases, either intentional or unintentional
Good quality data that meets the criteria outlined here helps AI models to make more precise predictions, which can lead to better outcomes and increased trust in the AI systems. Bad data, on the other hand, can start to surface all sorts of problems when it forms the foundation of an AI solution. Some of these problems include: inaccurate predictions, bias and discrimination, increased cost to find and fix data errors, negative user experience, legal and regulatory risks, and loss of trust.
Good data doesn’t happen by accident. It requires thoughtful process and governance controls to achieve. Without robust data governance practices such as clarity of data ownership, quality control processes, and data documentation, it becomes difficult to achieve the level of cleanliness required for accurate and ethical output from AI models. Security is equally important – in order to maintain data integrity and protect sensitive information, controls must be in place to protect from unauthorized access, tampering, or theft. Finally, data must be collected and used in an ethical and legal manner, respecting both privacy and consent regulations.
Data quality is an ongoing concern, and maintaining data quality often requires continuous monitoring, cleaning, and improvement efforts to ensure that data remains useful and reliable. Creating and maintaining comprehensive documentation is important as well, to help understand the source, meaning and history of data sets.
So what do you do if your data is currently in a less-than-ideal state? There are a number of steps that you can take, including running a data quality assessment, cleaning and pre-processing, and setting up a testing and validation strategy. The details of these is a bit beyond the intended scope of this article, but if you have questions or would like to find out more, get in touch with Trifecta and we can help you wade through your data challenges and get things to a state where you can being taking advantage of all of the rapid-fire advancements in the AI space.
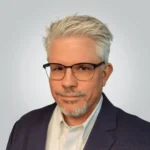